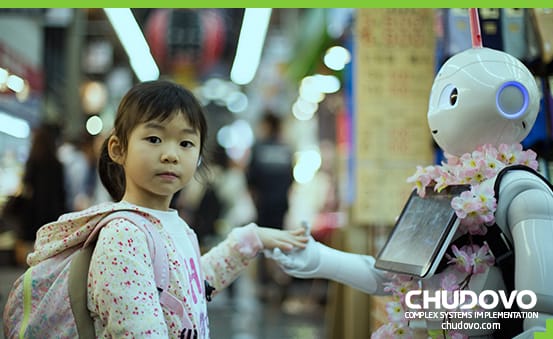
What Is Responsible Machine Learning?
The world is moving towards machine learning, becoming an evident and widespread option for developers and users today. In April 2021, Twitter introduced its responsible machine learning initiative. It was hardly a surprise because companies like Google and Microsoft had already taken steps towards compliant, secure, and human-centered artificial intelligence and ethics.
If you search around, you will find out that other companies have followed suit because ML and AI are being implemented in different products we use daily. With this responsible development and the use of advanced technologies, people will be able to carry out most of their business activities. And if responsible development of the technologies is not carried out, you could be affected in various ways like limited user experience or suffering from discrimination.
Tech companies globally should aim to engage in responsible machine learning. But first, there needs to be an understanding of what responsible machine learning is. In this article, we’ll discuss machine learning and its benefits and challenges.
Table of content
- Definition of responsible machine learning
- Principles that guide responsible machine learning
- Why you should care about responsible machine learning
- Benefits of responsible machine learning
- Challenges of responsible machine learning
- What’s responsible for AI
- Facets of responsible AI
- Benefits of responsible AI
- Conclusion
- FAQs
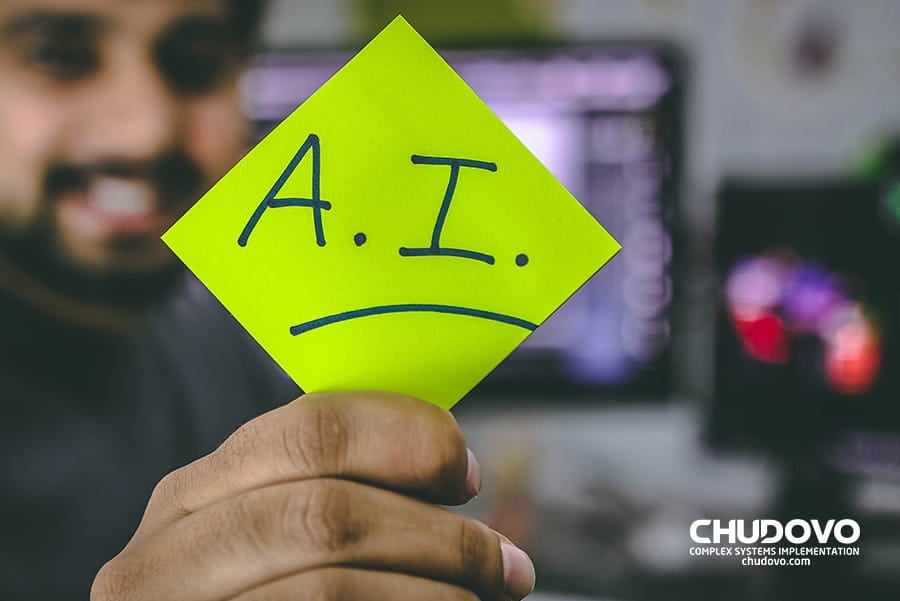
What Is Responsible Machine Learning?
Responsible machine learning is a set of principles and practices that ensure the ethical and responsible use of machine learning algorithms. This involves taking into account the potential risks and implications of using machine learning algorithms and taking steps to mitigate those risks. Responsible machine learning also involves being used in a way that is fair and equitable; an important part is ensuring that machine learning is used responsibly and ethically.
Arthur Lee Samuel– a pioneer in artificial intelligence research, defined machine learning as “a field of study that gives computers the ability to learn without being explicitly programmed.” The machine learns to think, act, and react through the essential instructions given to it. This tool even uses everything that has happened in the past to predict the future.
Today, advanced technology is disrupting every industry and even leading to innovations. Most of the services we use today, like Amazon, Twitter, Youtube, Google, Microsoft, Netflix, and beyond, use responsible machine learning.
If these services don’t use ML, you, as a user, could end up suffering from poor machine learning development and implementation. On the other hand, Twitter states that Machine learning involves responsibilities including taking care of its algorithm decisions, ensuring equality and fairness in its products, assuring transparency about all ml- related decisions, and enabling agency and algorithm possibility.
That said, there is no single definition of responsible machine learning. This is because different people, organizations, researchers, and industries have different meanings for responsible machine learning.
Principles that guide responsible ML
According to the institute of ethical AI and Machine learning, an organization that developed a series of principles to help guide the responsible development of machine learning, there are eight principles guiding responsible machine learning. They include:
1. Human augmentation
Human augmentation is the use of technology to enhance or improve human capabilities. This could include using prosthetics or exoskeletons to increase strength or using artificial intelligence to improve decision-making.
2. Bias Evaluation
This is the commitment to continually assess the potential for bias in a machine learning algorithm. It involves looking at the data used to train the algorithm, the algorithm itself, and its results to identify any potential sources of bias.
3. Explainability by Justification
If you are developing ML-based tools, always aim to improve transparency, explaining the decisions made by a machine learning algorithm. This involves explaining why the algorithm made certain decisions and how it arrived at them, making them understandable.
4. Reproducible operation
It is the ability of machine learning to produce consistent results when given the same input data. This is important for ensuring that the algorithm is reliable and trustworthy, as it ensures that the results are reproducible and can be verified. It helps to ensure that the algorithm is reliable and trustworthy.
5. Displacement strategies
Displacement strategies in machine learning refer to the techniques used to reduce the impact of automation on jobs and the workforce. These strategies include retraining and upskilling workers, providing financial support to those affected by automation, and creating new jobs in areas where automation has displaced workers.
6. Practical accuracy
This measures how well a machine learning algorithm performs in real-world scenarios. It differs from theoretical accuracy, which measures how well an algorithm performs on a given dataset, as it helps to ensure that the algorithm can make accurate predictions in real-world scenarios.
7. Trust by privacy
Trust by privacy is the practice of ensuring that data used to train a machine learning algorithm is kept secure and private. It involves using secure data storage and encryption techniques to protect the data, ensuring that only authorized personnel have access.
8. Data risk awareness
The data risk awareness principle covers every critical aspect of machine learning used for understanding the risks associated with using data to train machine learning algorithms. This involves understanding the potential for data bias, the potential for data leakage, and the potential for data misuse.
Following these principles, we could say that responsible machine learning is the act of developing and using machine learning algorithms to help humans while aiming to limit their negative impact by improving them through a thorough analysis of the technical structure and human factors.
Why You Should Care About Responsible Machine Learning
Why you should care depends on who you are. You could be a user, business owner, startup CEO, executive, manager, or even a developer working or learning about machine learning.
There are two ways to justify why you should care about responsible machine learning. The reason is easy to understand, especially if those principles can prevent your solutions from causing damage to users, team members, and even a company.
First, without proper guidance, machine learning can lead to discriminatory outcomes, incorrect insights, and even the disruption of entire processes. You might be a regular user, but you neither know the harm ML causes nor anything about machine learning solutions that are developed.
Secondly, as a user, you should care about responsible machine learning because it helps to ensure that the algorithms used to make decisions are fair, accurate, and secure. Responsible machine learning helps to ensure that the algorithms are not biased, that the data used to train the algorithms is secure and private, and that the results of the algorithms are reliable and trustworthy. Hence, responsible machine learning is essential for ensuring that machine learning algorithms are beneficial to society.
For companies, responsible machine learning helps to ensure that the algorithms used to make decisions are reliable. It involves ensuring that the data used to train the algorithms is secure and private and that the results of the algorithms are reproducible and can be verified.
Responsible machine learning is essential for ensuring that machine learning algorithms are beneficial to companies and their customers. Poorly developed and implemented machine learning algorithms can lead to offensive and discriminatory messaging, privacy breaches, and a poor customer experience.
Benefits of Responsible Machine Learning
Understanding the use cases and the benefits of responsible machine algorithms can determine if the algorithms are beneficial for both users and companies. Here are some benefits based on use cases:
Natural Language Processing
NLP is a branch of artificial intelligence that enables machines to understand and interpret human language. NLP is used in a variety of applications, such as text analysis, machine translation, and automated customer service. Responsible machine learning is essential for ensuring that NLP algorithms are beneficial to society, as it helps to ensure that the algorithms used to make decisions are fair, accurate, and secure and that the data used to train the algorithms is secure and private.
An example of machine learning is the chatbots that many organizations use to respond to customer inquiries on their websites. These chatbots are convenient as they are available 24 hours a day, allowing them to handle inquiries until human customer service agents become available.
This way, the human customer service agents aren’t overworked. And the company wouldn’t need to pay for those extra hours, hence, reducing costs.
Recognizing Images
Recognizing images in ML involves training algorithms to identify patterns in images and classify them. This facial recognition ability is potentially helpful for recognizing people in photographs and videos, as well as for security measures and product research.
For students, researchers, and the everyday user, image recognition helps them to find image references quickly and to research the places or persons in the photo. It’s exciting because years ago, you could only search with words, but now, people are searching with photos.
Data Mining
Data mining is the process of extracting useful information from large datasets. Machine learning can be used to automate this process, allowing algorithms to identify patterns and trends in data that will otherwise be difficult to detect. Data mining requires considerable processing power to allow the algorithm to identify trends in vast quantities of data, but it can help identify valuable patterns.
Better advertising and marketing
Machine learning can improve advertising and marketing in a company or industry by analyzing customer data. Machine learning algorithms can identify patterns and trends that can be used to target customers more effectively.
A responsible machine learning algorithm can predict the customers that will be interested in a particular product. This can help the company or user because the algorithm will have reliable information on customer behavior, which can help make the campaign more effective.
Developing better products
By analyzing customer data, machine learning algorithms can identify patterns and trends that can be used to create better products that meet customer needs. This way, product development isn’t a hit-or-miss situation. Businesses can have a higher chance of creating a hit but ethical, product with responsible machine learning.
Challenges of Responsible Machine learning
It is important to be familiar with the challenges of responsible machine learning to ensure that the algorithms used are beneficial to both users and companies. They include the following:
Lack of training data
Machine learning algorithms require large amounts of data to be trained effectively. And if there is not enough data available, the accuracy and reliability of the algorithm can be compromised.
Poor quality of data
This can lead to inaccurate results and can also lead to bias in the algorithm’s decisions. It is essential to ensure that the data used to train the algorithms is of high quality and that it is secure and private
Data overfitting
It occurs when an algorithm is trained on a dataset that is too small or when the algorithm is trained on a dataset that is too complex. This can lead to the algorithm making decisions that are not accurate or reliable, as it has not been trained on enough data to make accurate predictions.
Data underfitting
When the algorithm is trained on a dataset that is too simple or when the algorithm is not given enough data to make accurate predictions. This can lead to the algorithm making decisions that are not accurate or reliable.
Irrelevant features
These are features that are not relevant to the task at hand and can lead to the algorithm making decisions that are not accurate or reliable. Responsible ML requires providing explanations for why the algorithm made certain decisions and how it arrived at those decisions, but irrelevant features can muddle these explanations.
What’s Responsible AI?
Responsible AI is the practice of using artificial intelligence (AI) in a way that is ethical, secure, and beneficial to both users and companies. Successful business owners and organizations take a systematic approach to making responsible AI from scratch. They understand the complexity and scale of the change required.
Responsible Ai is an approach to developing, deploying, and assessing artificial intelligence in a safe, trustworthy way. It can help guide your decisions toward more beneficial and equitable outcomes.
The four pillars of responsible Ai include organizational, operational, technical, and reputational damage.
- Organizational damage refers to the potential for AI to cause harm to an organization’s reputation or financial standing.
- Operational damage refers to the potential for AI to cause operational disruptions or errors.
- Technical damage refers to the potential for AI to cause technical issues or security breaches.
- Reputational damage refers to the potential for AI to cause damage to an organization’s reputation or public image
Facets of Responsible AI
Organizations working with AI should follow several fundamental principles to ensure their technology is developed and used in a socially responsible way. They are;
- Fair – Fairness requires that the algorithms used to make decisions are unbiased and do not discriminate against any group of people
- Transparent – Transparency requires explaining why the algorithm made certain decisions and how it arrived at them.
- Accountable – Accountability requires that organizations are held responsible for the decisions made by their AI.
- Accurate – Accuracy requires that the algorithms are reliable and make decisions that are accurate and consistent.
- Secure – Security requires that the data used to train the algorithms is secure and private.
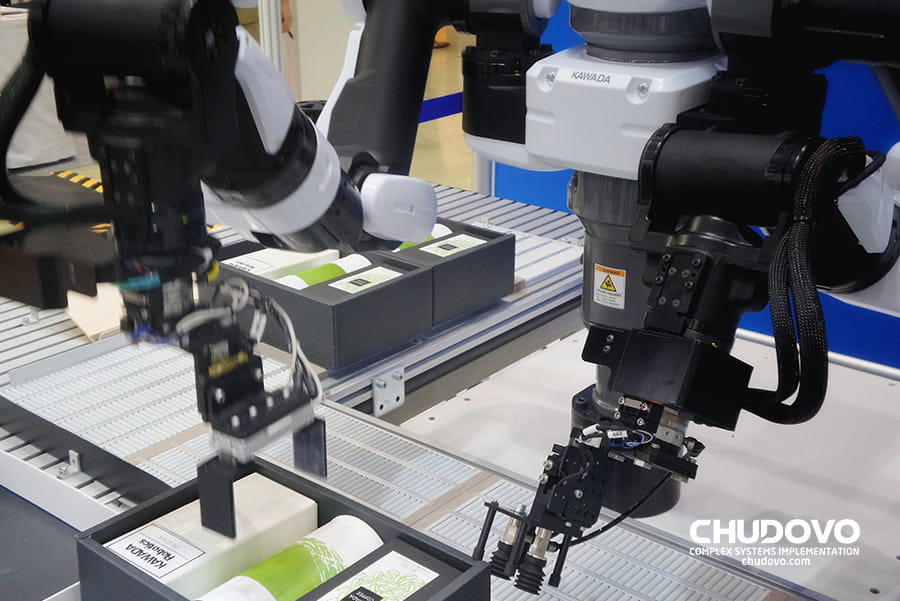
Benefits of Responsible AI
There are different benefits of using Ai responsibly in a business or organization. Let’s discuss them;
Automation
This is one of the most common benefits of AI. Automation is using technology to automate tasks that would otherwise be done manually.
Automation can be used to improve efficiency and accuracy, as well as reduce costs. Businesses can also use AI to reduce human oversight, as AI algorithms can be used to make decisions without the need for human intervention.
Smart decision-making
This is the process of using data and AI algorithms to make decisions that are accurate, reliable, and beneficial. AI can be used to coordinate data delivery, create data consistency, analyze trends, quantify uncertainties, and provide forecasts to help make the best decisions for a company.
Good customer experience
AI-powered solutions can help businesses with responding to messages, by providing personalized recommendations, automated customer service, and predictive analytics. Customers will be satisfied and return more frequently.
Medical Advances
AI can be used to diagnose diseases, develop treatments, and improve patient outcomes. Overall, it’ll improve service delivery in medicine.
Solving complex user and business problems
Identifying and solving user and business problems can be done through the use of AI. AI can be used to identify patterns, analyze data, and make decisions that are accurate and beneficial.
Minimizing error
The robotic automation tool can ensure that the algorithms used to make decisions are accurate and reliable and that the data used to train the algorithms are of high quality are relevant to the problem being solved. This reduces the total error in predictions and results. Hence, businesses and users will be more confident in that decisions made using AI data.
Managing repetitive tasks
For anyone, it takes a great level of effort and concentration to stick to and execute recurring tasks. The good thing is that responsible artificial intelligence can help. Businesses can use Artificial Intelligence to automate tasks that would otherwise be done manually, improving efficiency and accuracy
Business continuity
AI-powered chatbots and virtual assistants offer several advantages, including reduced reliance on human agents, increased operational efficiencies, and improved customer experience. These benefits are especially valuable to businesses during times of crisis, as they can help to reduce costs and improve customer service. Additionally, AI-powered chatbots and virtual assistants can provide personalized recommendations and automated customer service, which can help to improve customer satisfaction.
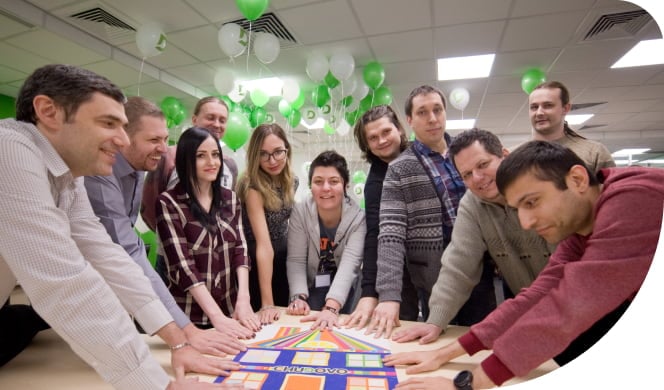
Certified engineers
Convenient rates
Fast start
Profitable conditions
Agreement with
EU company
English and German
speaking engineers
Conclusion
Responsible machine learning should be the go-to goal for everyone, as Machine learning will affect all of us at some point in our daily life, including trying to buy something from your favorite online website. Artificial intelligence, applied through machine learning, is one of the most transformational technologies of this generation, helping to tackle some of humanity’s most challenging problems and maximize productivity. You’ll need to embrace it, discuss it, and monitor it.
In conclusion, machine learning is a powerful tool for automating tasks, making decisions, and solving complex problems. Responsible machine learning requires stakeholders to ensure that the algorithms used to make decisions are fair, accurate, and secure and that the data used to train the algorithms is factual and private.
FAQs
What is the specific problem that responsible machine learning can solve?
The specific problem that a responsible machine learning model can solve is to accurately and reliably identify patterns in data that can be used to make decisions that are beneficial to the user or business. According to Alyssa Simpson Rochwerger and Wilson Pang, authors of the book “Real World AI: A Practical Guide for Responsible Machine Learning,” just 20% of artificial intelligence system pilots deployed by major companies advance to production. Furthermore, those that do often fail to efficiently serve their customers.
How can you update and maintain your machine learning model to ensure it’s adaptable?
To maintain and update your model to ensure it is adaptable, you need to regularly monitor the performance of the model and adjust it as needed. This can include adding new data, adjusting the parameters of the model, or retraining the model with new data. Additionally, it is important to ensure that the data used to train the model is of high quality and relevant to the problem being solved. Finally, it is important to ensure that the model is tested regularly to ensure that it is performing as expected.
Who are the experts who will oversee the development of a machine-learning model?
The experts who will oversee the development of any machine-learning model will depend on the specific problem being solved. Generally, experts in the field of data science, machine learning, and artificial intelligence will be needed to ensure that the model is developed correctly and can accurately and reliably solve the problem. Additionally, data security and privacy experts may be needed to ensure that the data used to train the model is secure and private.
So what are you still waiting for? Following this link to learn more about our machine learning services.